Mikhail Zhilkin: How to hire your first data scientist
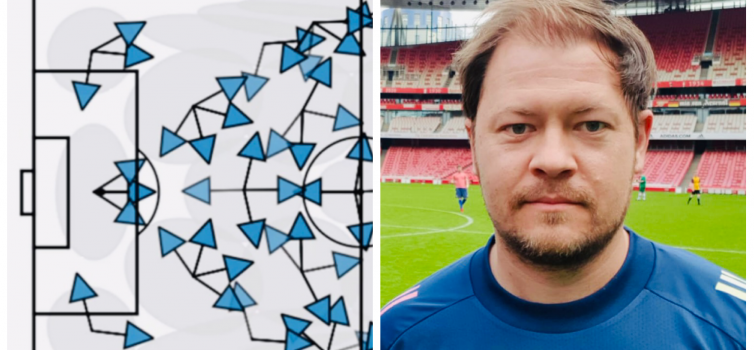
Mikhail Zhilkin is a data scientist with Arsenal
Written by Mikhail Zhilkin — November 2, 2021
INDUSTRIES such as IT and finance are years ahead of football in terms of using data to get an edge over the competition.
Take Candy Crush, a popular franchise of free-to-play mobile games that I worked on for four years before joining Arsenal in 2018. It is tended to by a double-digit number of data scientists and engineers.
With tens of millions of daily users, it’s a logistical and technological challenge to ensure that the data of users’ activity is collected, stored and piped into numerous reports and dashboards. The amount and depth of data collected enables sophisticated analysis of player behaviour and automated content generation.
In football, however, data analytics is a relatively new branch of operations. Most clubs and national federations fall into one of the two categories:
- There are no dedicated data analysts and data is used sparingly, if at all.
- There are a small number of people working with data, and the club/ federation are still exploring ways to benefit from their efforts.
When an organisation is new to data analytics, the most important component of success is people. If a data-mature company miss-hires its umpteenth data scientist, it won’t be the end of the world.
Surrounded by experienced colleagues, with best practices and processes in place, an incompetent person may do only so much damage. In contrast, when a football club hires their first ‘data person’, his or her incompetence can slow down the adoption of data analytics for months or even years.
A training ground is full of people who can test your knowledge of the game, of sports medicine, of exercise science, and so on. There are, however, few people eager to debate database architecture, statistical methods or writing code.
It is easy for a budding data science team to go astray and become one of the following things:
- A toy. In the days of the dot-com bubble, everyone wanted a website. Whether the website did anything for the business was irrelevant. Data science is the new dot-com website: it has potential to be useful, but it’s not enough to just have it - it must serve a business goal. In football, that’s winning games.
- A marketing tool. ‘Big data’, ‘machine learning’ and ‘AI’ look good in PowerPoint presentations, but what is often missing is how any of these actually change anything. In that situation, data science is just a shiny wrapper filled with hot air.
- A decision-justifier. This is the hardest one to call out. It may look perfectly legit: data, analytics, reports and dashboards. Everything is there, and it’s all being looked at by decision-makers. But when a decision is backed by the data, people don’t necessarily stop to ask, “Would we have acted differently if the numbers had been different?” If the answer is no, then data science has been nothing but a ritual to justify the decision that had already been made. To quote from my recently-published book, Data Science Without Makeup, “The end goal of data science is to change opinions.”
When it comes to data analytics, the first hire is as make-or-break as it gets. So how do you find and hire the best candidate?
A common misconception is that in order to work in football, you need football-related experience and qualifications. This may be largely true for coaches and analysts, but less so for data people.
A data scientist working at a football club can quickly pick up what they need to know about the rules of the game, tactics and the physical aspects. In contrast, someone with loads of football experience but insufficient data skills will struggle.
If your job ad makes it clear that data skills are all-important and that knowledge of football only a bonus point, you won’t risk pushing away perfectly suitable candidates.
To give yourself a chance of hiring someone good, you obviously need to make sure good candidates apply in the first place. Many people are passionate about football, but it’s unlikely that an experienced data scientist will take a significant pay cut just to work in the sport.
A low-paid position may attract passion, but not competence. Passion may be enough for an internship, but not for someone who will be laying down the foundation of data analytics at a club.
Offering a competitive salary will hopefully attract a few good candidates, so the next challenge is to pick them out. Unless the club already have a data scientist that they fully trust, the judgment will be made by a person or people with limited understanding of the field.
Beyond basic screening, a CV is mostly indicative of how good the candidate is at composing a CV. I used to be part of the recruitment process at King, the makers of Candy Crush, and my main takeaway was that CVs are a poor predictor of how an interview will go.
A candidate with a mediocre CV could turn out to be very capable and keen and we would have an engaging conversation, while someone with an impressive CV full of achievements could make me think, “If I can barely endure a 45-minute conversation with this person, I cannot imagine it being good to have them as a full-time colleague.”
Technical skills are always best judged in action. You probably already have some data from games or the training process (failing that, there are publicly available data sets) and questions you’d like to answer using data (otherwise, why are you looking for a data scientist?) so you have enough to offer candidates a take-home test.
This doesn’t have to be hard. It can be as simple as calculating the total distance each player covered from raw tracking data and plotting it as a bar chart.
The best book on recruitment I’ve read is “Smart and Gets Things Done”, and the title alone sums it up nicely. The purpose of the take-home test is exactly that - to check if the candidate is smart enough to solve a typical problem, and that he or she gets things done.
Talking to the candidate about their experience working on real-life projects is another opportunity to verify that their work translates into a tangible result. A fraction of applicants will send the take-home test back and you’ll hopefully be left with a handful of candidates you can invite for an in-person interview.
There are two more things you want to focus on when interviewing for a position in football:
- Does the candidate understand what the job will entail and are they okay with it? Football is an unusual industry, and people may have all kinds of ideas. If you’re looking for someone who can take charge of spreadsheets and replace them with automated reports, you need someone who’s ready to do just that and doesn’t expect to be in charge of the starting eleven.
- Can the candidate explain complex things in a simple way? A data scientist at a football club will mostly speak to people without a STEM degree. If he or she cannot make their work transparent to the end-users, it will limit their impact even if they have best intentions. And if they don’t have the best intentions, complexity is the best way to cover up bullshit.
If you find these pointers useful or even intellectually stimulating, you may be interested in reading my book, Data Science Without Makeup, in which data science recruitment and other topics are explored in detail. Many of the examples used come from my three years (and counting) as a data scientist at Arsenal.